Watchful Orbs: How AI Could Transform Healthcare
January 6, 2024 | Abdus-Salaam Muwwakkil - Chief Executive Officer
Table of Contents
- Introduction
- Safety and Risk Considerations
- Basic Assumptions and Framework
- Clinical Communication and Documentation
- Transforming AD/ADRD Care Through AI Documentation
- Transforming Hospital Discharge
- Rethinking Medication Reconciliation
- Patient Understanding and Access
- Healthcare Economics
- Healthcare Equity and Access
- Addressing Common Concerns
- From Vision to Reality: The Technical Foundations
- Conclusion: The Moral Imperative
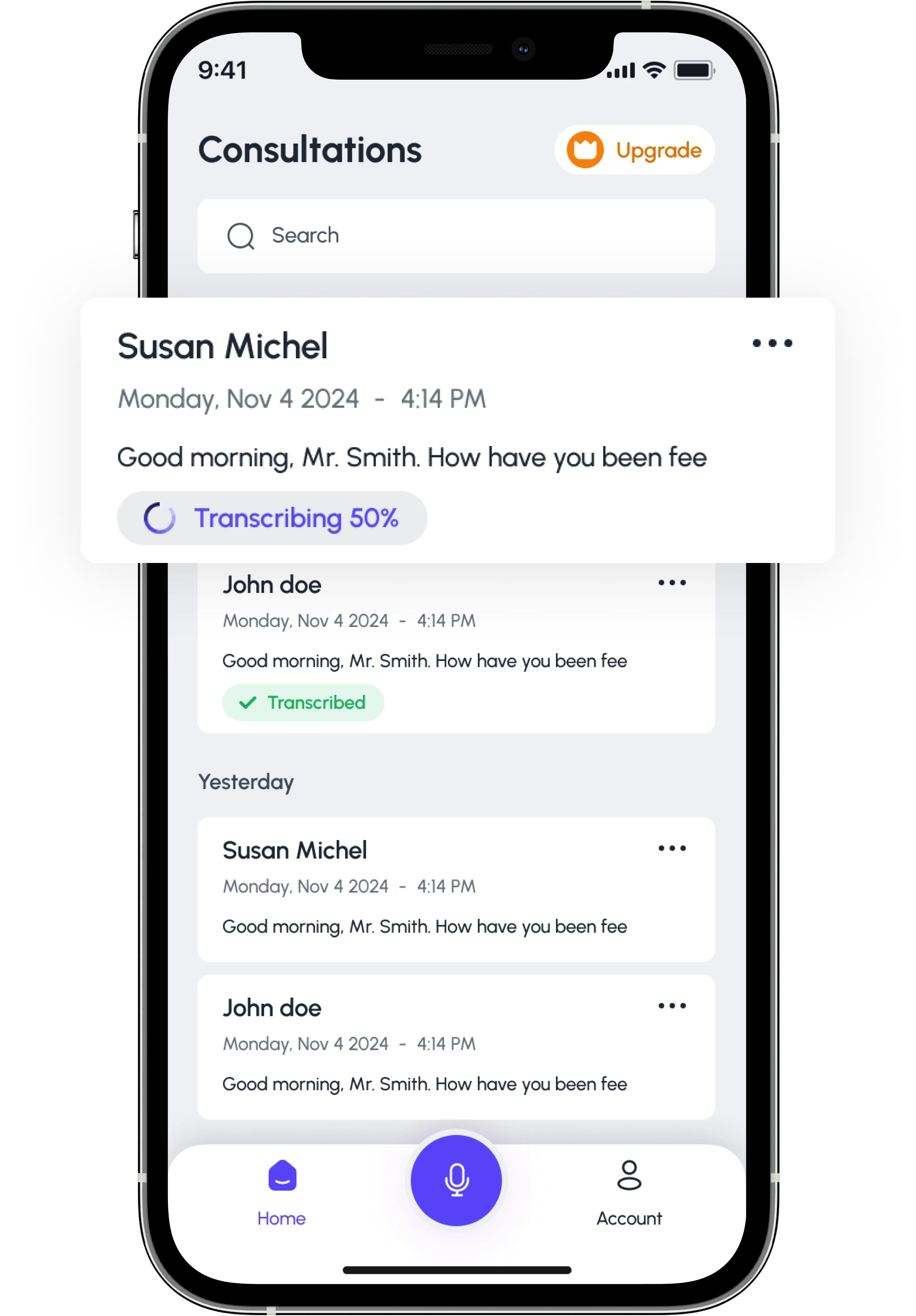
Introduction
I think and talk a lot about the risks of AI in healthcare documentation. Our team at OrbDoc spends significant time researching how to make clinical voice AI safe, accurate, and reliable. Because of this focus on safety and validation, people sometimes conclude that I’m skeptical about AI’s role in healthcare or that I see mainly dangers ahead. I don’t think that at all. In fact, my intense focus on safety and thoughtful implementation stems from believing these are the main obstacles between us and a fundamentally transformative future for healthcare delivery.
In this essay, I try to sketch out what that future might look like—how healthcare could function if we get AI-enabled clinical documentation right. Of course, predicting healthcare’s future is notoriously difficult; the field has proven remarkably resistant to technological disruption, and the effects of AI may be even more unpredictable than past innovations. So while this vision is unavoidably speculative, I’m aiming for educated and useful speculation that captures the essence of what’s possible, even if many specific details prove wrong. I include concrete technical details because I believe a tangible vision does more to advance discussion than abstract possibilities.
Safety and Risk Considerations
First, I want to explain why those of us working on clinical AI often seem to focus primarily on risks and safety. This emphasis stems from several key considerations:
-
Immediate Human Impact: Healthcare mistakes have immediate human impact. Unlike many domains where AI is being deployed, errors in healthcare can directly harm people. When we talk about the promises of AI, we must first establish that we understand and respect this fundamental reality of healthcare innovation.
-
Earning Trust: Trust must be earned systematically. Healthcare has a long history of technologies that promised transformation but created new burdens instead. The medical community’s skepticism is earned and valid. Building trust requires first demonstrating that we understand not just the technical challenges, but the complex human and systemic factors at play.
-
Hype Can Be Harmful: When healthcare technology companies oversell capabilities or understate risks, it can lead to dangerous implementations and erode trust in genuine innovations. We’ve seen this pattern repeatedly with EHRs, clinical decision support, and early AI systems. The result isn’t just failed technology—it’s increased burden on already strained healthcare professionals.
-
“Do No Harm”: Healthcare’s first principle isn’t “move fast and break things”—it’s “first, do no harm.” This ethical foundation shapes how we must approach innovation in this space, demanding rigorous validation and careful implementation.
Yet despite these important cautions, I really do think it’s critical to articulate what transformed healthcare documentation could look like, while doing our best to avoid the pitfalls above. In fact, I believe having a genuinely inspiring vision of the future is essential, especially in healthcare where burnout and cynicism about technology run high. Many aspects of healthcare technology can feel adversarial or burdensome, but ultimately, there has to be something we’re working toward, some positive-sum outcome where everyone—patients, providers, and the healthcare system itself—is profoundly better off.
While the list of potential applications for AI in healthcare is extremely long, I’m going to focus on five areas that seem to have the greatest potential to directly improve care delivery:
- Clinical Communication and Documentation: transforming how healthcare professionals capture and share information
- Patient Understanding and Access: making healthcare knowledge truly accessible to those who need it
- Healthcare Economics: ensuring resources flow efficiently to support optimal care
- Clinical Decision Support: augmenting rather than replacing clinical judgment
- Healthcare Equity and Access: extending quality care to underserved populations
Key perspective: My predictions may seem radical by most standards, but I mean them earnestly and sincerely. Everything I’m saying could easily prove wrong, but I’ve attempted to ground my views in careful analysis of how clinical workflows might be transformed and what that could mean in practice.
My experience building voice AI systems for clinical settings informs these views, but I’m certain I’ll get plenty wrong. This essay is best viewed as an invitation to imagine and discuss healthcare’s AI-enabled future, not as a definitive prediction.
Basic Assumptions and Framework
To make this vision more concrete and grounded, let’s establish what we mean by advanced clinical AI, as well as a framework for thinking about its implementation and impact in healthcare settings.
Beyond Simple Language Models
First, this won’t be about generic language models in healthcare or simple voice transcription. When I talk about advanced clinical AI, I’m describing something far more sophisticated. These systems will understand medical conversation at the level of an experienced clinician, grasping complex contexts, subtle implications, and critical details that might be missed by current systems. They won’t just passively transcribe but will actively synthesize information across an entire patient stay, connecting insights from multiple providers and timeframes.
Technical insight: What makes these systems truly transformative is their seamless integration with existing hospital systems while maintaining rigorous security and privacy standards.
They’ll operate at significantly faster than human speed while maintaining accuracy, potentially processing hundreds of patient encounters simultaneously. Most importantly, they’re mobile-first and voice-native, designed to fade into the background of clinical work rather than demand attention.
The timeline for such systems might seem aggressive to some and conservative to others. Based on current trajectories in both AI and healthcare technology adoption, I expect initial versions within 2-3 years, with mature implementations in 5-7 years. However, the path to this future isn’t straightforward, and understanding the limiting factors is crucial.
Limiting Factors in Healthcare
Healthcare faces unique constraints that affect how quickly and effectively AI can be deployed. I think about these in several distinct but interrelated categories.
Physical World Constraints
Critical consideration: Unlike many AI applications, clinical documentation happens in the physical world of hospital wards and clinic rooms.
Doctors and nurses move constantly, interact with patients face-to-face, and work in environments with strict protocols and procedures. Any AI system must respect these physical realities. A solution that requires clinicians to stand still at a workstation or break patient eye contact won’t succeed, no matter how technically sophisticated it is.
Data Constraints
Healthcare data isn’t just complex—it’s heavily regulated and often fragmented. HIPAA compliance isn’t optional, and interoperability challenges that have plagued healthcare for decades don’t magically disappear with AI. Moreover, medical knowledge is vast and constantly evolving. Training AI systems requires not just large amounts of data but also high-quality, well-structured data that captures the nuances of clinical practice.
Human Factors
Core principle: Healthcare is fundamentally human. Patient trust, clinical judgment, and the doctor-patient relationship cannot be compromised.
Healthcare professionals have developed sophisticated workflows over decades, and changes must enhance rather than disrupt these patterns. The “returns to intelligence” in healthcare are actually limited by human factors more than technical ones.
Regulatory Requirements
Healthcare innovation operates within a complex regulatory framework designed to protect patient safety. While this can slow adoption, it’s not just red tape—it reflects healthcare’s core value of “first, do no harm.” Any AI system must navigate these requirements thoughtfully.
A Framework for Impact
Strategic framework: Given these constraints, how should we think about AI’s potential impact on healthcare? I propose evaluating potential transformations through three critical lenses.
-
Time to Impact
- Some changes can happen quickly once the technology is ready (like improving transcription accuracy)
- Others require systemic changes and will take longer (like reducing readmission rates through better care coordination)
-
Depth of Impact
- Some changes are incremental improvements to existing processes
- Others fundamentally transform how care is delivered
- Both matter, but distinguishing between them helps set realistic expectations
-
Prerequisites for Success
- What needs to happen—beyond just technical capability—for each transformation to succeed?
- This might include regulatory changes, workflow adaptations, or cultural shifts in healthcare organizations
Clinical Communication and Documentation
Startling reality: The numbers are stark: doctors currently spend two hours on documentation for every hour they spend with patients.
In a typical hospital stay, critical information is scattered across dozens of notes from different providers, written at different times, often in different systems. This fragmentation isn’t just inefficient—it’s dangerous. Studies show that poor documentation contributes to over 5 million preventable medical errors annually, resulting in more than 100,000 deaths. When critical information gets lost between shifts or providers, patients suffer.
The Documentation Crisis
What’s particularly striking about this crisis is that it’s relatively new. The documentation burden has actually increased significantly with computerization. Electronic Health Records (EHRs), for all their benefits, have often made documentation more time-consuming, not less. This isn’t just ironic—it’s a crucial lesson about how technology needs to adapt to healthcare, rather than forcing healthcare to adapt to technology.
Timeline perspective: I believe AI could effectively solve this crisis within 5-7 years, but only if we understand what “solving” really means.
It’s not just about faster typing or better transcription. The goal isn’t to help doctors write more notes—it’s to fundamentally transform how clinical information flows through the healthcare system.
A Vision of Transformed Documentation
Let me paint a picture of what this transformation might look like:
Imagine a resident physician starting her morning rounds. As she enters each patient’s room, her mobile device is already listening, understanding, and contextualizing every interaction. She speaks naturally with patients and nurses, never breaking eye contact to type or click. The AI doesn’t just transcribe—it understands the clinical significance of every conversation, automatically updating the patient’s record with relevant information while filtering out irrelevant details.
When she mentions a new symptom, the system automatically cross-references it with the patient’s history and recent medications. When she discusses treatment changes, these are automatically reconciled with existing orders and checked for potential conflicts. If she needs to reference something from previous notes, she can simply ask aloud, and the AI provides relevant context immediately.
Key feature: Most importantly, this all happens in real-time, on a mobile device, without requiring her to break clinical workflow or patient interaction. The technology fades into the background, enhancing rather than interrupting the practice of medicine.
Beyond Individual Interactions
But the impact goes far beyond individual doctor-patient interactions. Here’s what becomes possible:
Seamless Care Transitions
When the night shift takes over, they don’t have to hunt through dozens of notes to understand what happened during the day. The AI has already synthesized the key information, highlighting important changes and maintaining continuity of care. This alone could significantly reduce handoff errors, which currently affect nearly 1 in 5 patients.
Real-time Quality Measures
Instead of retrospectively reviewing charts for quality metrics, hospitals can track care quality in real-time. The AI understands not just what was documented, but what it means clinically, enabling immediate feedback and intervention when needed.
Enhanced Clinical Collaboration
When a specialist is consulted, they don’t start from scratch reading through days of notes. They receive a precise, contextual summary of relevant information, automatically generated and continuously updated.
Predictive Care Insights
By understanding the patterns in patient progression over time, the system can flag subtle changes that might indicate deterioration before it becomes obvious. This isn’t just about individual data points—it’s about understanding the narrative of each patient’s care.
Technical Requirements and Progress
Current capabilities: The technical requirements for this vision are significant but achievable. We’re already seeing early versions of these capabilities:
- Voice recognition accuracy in medical contexts has reached 98%
- Natural language understanding can now reliably extract clinical concepts from conversation
- Mobile devices have the processing power to handle complex AI tasks locally
- Graph neural networks can maintain context across multiple conversations and documents
Real-World Challenges
But the real challenges aren’t primarily technical. They involve:
- Integrating seamlessly with existing hospital workflows
- Maintaining privacy and security in mobile contexts
- Building trust with healthcare providers
- Navigating regulatory requirements
- Ensuring equitable access to these technologies
Implementation timeline: This is why I believe a 5-7 year timeline is realistic but not conservative. The core technology might be ready sooner, but full implementation requires careful attention to these human and systemic factors.
Transformative Implications
The implications of getting this right are profound. Beyond the obvious efficiency gains, think about what it means for medical education, for patient safety, for provider satisfaction. When documentation becomes effortless and accurate, doctors can focus entirely on patients. When information flows seamlessly between providers, care becomes more coordinated and effective. When clinical patterns become immediately visible, medical knowledge itself can advance more quickly.
Paradigm shift: This isn’t just about making existing processes more efficient—it’s about enabling entirely new ways of delivering care.
And importantly, unlike many technological advances in medicine’s history, this one doesn’t require doctors to change how they practice. Instead, it adapts to them, enhancing rather than disrupting the essential human elements of healthcare.
Transforming AD/ADRD Care Through AI Documentation
The challenges we’ve discussed in healthcare documentation take on particular urgency in the context of Alzheimer’s Disease and related dementias (AD/ADRD). With 6.9 million Americans living with these conditions and that number projected to double by 2060, we face a critical inflection point: either documentation continues to burden an already strained system, or we transform how we capture and utilize clinical information in AD/ADRD care.
Why AD/ADRD Demands Special Attention
The documentation challenges in AD/ADRD care are uniquely complex. Unlike many other medical conditions, AD/ADRD requires capturing subtle cognitive changes over time, coordinating across multiple care settings, and managing complex transitions that often involve both medical and social support systems.
Critical statistic: When 70% of caregivers report significant stress in coordinating care, we’re not just seeing a documentation problem – we’re seeing a human crisis that documentation failures make worse.
Current documentation practices particularly struggle with three critical aspects of AD/ADRD care:
-
Cognitive Assessment Documentation First, cognitive assessments require precise documentation across multiple domains. Tools like the Montreal Cognitive Assessment (MoCA) demand careful attention to detail, yet providers often must choose between maintaining patient engagement and thorough documentation. This trade-off becomes more acute as cognitive decline progresses.
-
Complex Care Transitions Second, care transitions in AD/ADRD settings involve extraordinary complexity. Each transition requires communicating not just medical information, but details about cognitive status, functional abilities, and social support systems. When documentation fails to capture these nuances, we see the results in preventable readmissions and fragmented care.
-
Progressive Condition Monitoring Third, the progressive nature of AD/ADRD means that subtle changes in patient status can have profound implications for care. Documentation systems designed for episodic care often fail to capture these gradual shifts, making it harder to adjust care plans proactively.
Reimagining Documentation for AD/ADRD Care
Vision: The transformation of clinical documentation through AI offers particular promise for AD/ADRD care settings.
Here’s how this might look in practice:
Imagine a memory care unit where providers can focus entirely on patient interaction during cognitive assessments. The AI system doesn’t just transcribe – it actively structures information according to standardized assessment frameworks, flagging subtle changes from previous evaluations. Rather than wrestling with documentation templates, providers can maintain the crucial human connection that makes accurate assessment possible.
During care transitions, the system would automatically synthesize relevant information from multiple sources, creating comprehensive yet focused handoff documents that highlight key cognitive and functional status changes. This isn’t just about preventing errors – it’s about enabling truly coordinated care across settings.
Key benefit: Most importantly, this transformation would support the central role of caregivers in AD/ADRD care. When 60% of facilities struggle to help families navigate care effectively, better documentation could provide the clarity and continuity that caregivers desperately need.
Technical Reality and Human Impact
While the technology for this transformation is rapidly evolving, we must acknowledge current limitations. Speech recognition in clinical settings still shows error rates around 7.4%, and capturing the nuances of cognitive assessment requires advances in both accuracy and contextual understanding.
Yet the stakes are too high to wait for perfect solutions. Even incremental improvements in documentation accuracy and completeness could significantly impact care quality. When we consider that each documentation error in AD/ADRD care can cascade into serious care coordination problems, the value of progress becomes clear.
The Path Forward
The path forward requires balancing technical innovation with practical implementation. This means:
-
Adaptive Systems Development
- Systems that adapt to the specific challenges of AD/ADRD care settings
- Handling variable patient communication abilities
- Managing complex care coordination needs
-
Documentation Framework Evolution
- Creating frameworks that support standardized assessment tools
- Maintaining flexibility for individual patient needs
- Supporting longitudinal tracking of cognitive changes
-
Human-Centered Design
- Ensuring technology enhances rather than disrupts crucial human elements
- Supporting caregiver involvement and communication
- Maintaining dignity and respect in patient interactions
Looking Ahead
Core mission: The transformation of AD/ADRD care documentation isn’t just about technology – it’s about fulfilling our fundamental obligation to provide the best possible care for an increasingly vulnerable population.
As we work to realize this vision, we must remember that success will be measured not in technical metrics alone, but in reduced caregiver stress, better care coordination, and improved quality of life for those living with AD/ADRD.
This isn’t just another healthcare technology initiative. It’s an opportunity to fundamentally transform how we support some of our most vulnerable patients and their caregivers. The technical challenges are significant, but the human imperative makes this transformation essential.
Transforming Hospital Discharge
Crisis point: The crisis in hospital discharge documentation represents a particularly stark example of how our current healthcare tools fail both providers and patients.
Consider this reality: 88% of discharge instructions are written at a level patients cannot understand, and nearly 40% of patients leave the hospital without fully grasping their diagnosis. This isn’t just a documentation problem—it’s a human crisis that costs the healthcare system $52 billion annually in preventable readmissions.
What makes this particularly striking is that the challenge isn’t primarily medical—it’s informational. The knowledge exists in the hospital system, scattered across hundreds of notes, lab results, and medication orders. But synthesizing this information into a coherent narrative that serves both clinical and patient needs has proven remarkably difficult. Current approaches often task the most junior doctors with this crucial responsibility, leading to predictably inconsistent results.
Beyond Simple Summarization
Transformation timeline: I believe AI could fundamentally transform this aspect of healthcare within 3-5 years, but only if we understand what real transformation looks like.
It’s not just about automated summarization or simplifying medical language. The goal isn’t to help doctors write better summaries—it’s to fundamentally reshape how information flows from the hospital to all stakeholders in a patient’s care.
Let me paint a picture of what this transformation might look like:
Imagine a system that doesn’t just passively collect information but actively synthesizes the entire hospital stay in real-time. As different providers interact with a patient over multiple days, the AI builds a comprehensive understanding of the case, linking related information across time and maintaining the crucial context that often gets lost in traditional documentation.
Key innovation: When discharge time approaches, this system doesn’t just summarize—it creates multiple synchronized versions of the discharge information, each tailored to its audience.
- For receiving physicians, it generates a precise clinical summary that preserves the sophisticated medical context they need
- For patients, it creates clear, actionable instructions at a sixth-grade reading level in their preferred language
- For family caregivers, it provides practical guidelines that account for their specific circumstances
The Technical Foundation
The technology to enable this transformation exists today. Through advanced neural networks and knowledge graphs, we can now maintain complex temporal relationships across multi-day stays, understanding not just what happened but how events relate to each other. Natural language processing can achieve 99% accuracy in medical contexts, while graph-based architectures can track intricate relationships between different aspects of care.
Core capability: But the real innovation isn’t in language processing—it’s in context management.
By treating the hospital stay as a continuous narrative rather than a series of disconnected notes, we can capture the subtle patterns and connections that make medical care coherent. When a new symptom appears, the system automatically links it to relevant past events. When treatments change, it maintains a clear record of the medical reasoning involved.
Real Impact on Care
Recent studies validate this approach. AI-generated discharge summaries achieve better compliance with clinical standards than those written by junior doctors, while simultaneously scoring dramatically better on patient comprehension measures. But the impact goes far beyond quality metrics.
Think about what this means for care transitions. When a primary care physician receives a new patient post-discharge, they don’t have to piece together the hospital course from fragmented notes. Instead, they get a coherent narrative that preserves all the crucial medical context while highlighting the key decisions and changes in the patient’s condition.
More importantly, think about what this means for patients. When discharge instructions are truly understandable—when patients leave the hospital confident in their ability to manage their care—we don’t just reduce readmission rates. We fulfill healthcare’s fundamental promise to actually transfer knowledge and agency to the people we serve.
Impact metric: Studies suggest we could reduce readmission rates by 25-30% through better discharge communication alone.
But the human impact goes far beyond these statistics. We’re talking about transforming one of healthcare’s most critical transitions—the moment when institutional care must translate into successful self-management.
This isn’t just about documentation efficiency—it’s about using AI to solve one of healthcare’s most persistent challenges: the gap between the sophisticated care we deliver in hospitals and patients’ ability to maintain that care at home. When we get this right, we’re not just improving healthcare delivery—we’re fundamentally enhancing its ability to achieve its core mission.
Rethinking Medication Reconciliation
Among healthcare’s many documentation challenges, medication reconciliation stands out as both critically important and stubbornly resistant to current solutions. The statistics are sobering: medication errors affect millions of patients annually, with a significant portion occurring during care transitions. What makes this particularly frustrating is that many of these errors stem not from lack of medical knowledge but from our inability to maintain accurate, comprehensive medication information across time and settings.
Core challenge: The challenge appears deceptively simple: maintain an accurate list of a patient’s medications throughout their care journey.
But in practice, this involves tracking complex temporal relationships, understanding intricate medication interactions, and synthesizing information from multiple providers and systems. During a typical hospital stay, a patient’s medications might be adjusted dozens of times, with each change rippling through their entire treatment plan.
Beyond List Management
Timeline perspective: I believe AI could transform medication reconciliation within 3-5 years, but only if we fundamentally rethink our approach.
It’s not enough to simply digitize medication lists or automate current processes. We need systems that understand medications in their full clinical context—not just what drugs a patient is taking, but why they’re taking them, how they interact, and how they relate to the overall care plan.
Imagine a system that builds a living model of a patient’s medication journey using advanced graph structures and knowledge networks. As medications change during a hospital stay, the system doesn’t just record the changes—it understands their implications, tracks their relationships to other aspects of care, and maintains a clear picture of the patient’s medication status at any point in time.
When a new medication is prescribed, the system automatically cross-references it with the patient’s entire medication history, known interactions, and clinical context. When doses are adjusted, it tracks not just the changes but the medical reasoning behind them. This isn’t just about avoiding errors—though that alone would justify the transformation. It’s about enabling a fundamentally more intelligent approach to medication management.
The Technical Foundation
The technology to enable this transformation exists today. Through graph neural networks and medical knowledge graphs, we can now model complex medication relationships and their evolution over time. Natural language processing can extract medication information from clinical conversations with high accuracy, while temporal reasoning systems can track medication changes across a patient’s entire stay.
Key innovation: But the real innovation isn’t in the individual technologies—it’s in how they work together to create a comprehensive understanding of a patient’s medication journey.
By treating medications not as isolated prescriptions but as part of an interconnected network of clinical decisions, we can capture the subtle relationships and patterns that make medication management coherent and safe.
Impact on Care Quality
The implications of getting this right extend far beyond simple documentation. When medication reconciliation becomes truly intelligent, it transforms from a documentation burden into a powerful tool for clinical decision support. Doctors can instantly understand a patient’s complete medication history, including past reactions, interactions, and treatment patterns. Care transitions become safer and more efficient, with receiving providers getting clear, comprehensive medication information that preserves all crucial context.
Patient benefit: Most importantly, patients leave the hospital with clear, accurate medication instructions that take into account their entire medication history and specific circumstances.
This isn’t just about avoiding errors—it’s about enabling patients to become active, informed participants in their medication management.
Studies suggest that improved medication reconciliation could prevent hundreds of thousands of adverse events annually. But the impact goes beyond safety metrics. By transforming one of healthcare’s most complex and error-prone processes into a source of clinical insight, we’re not just avoiding mistakes—we’re enabling better care decisions.
This transformation in medication reconciliation exemplifies the broader potential of AI in healthcare: not just automating existing processes, but fundamentally enhancing our ability to deliver safe, effective care. When we get this right, we’re not just solving a documentation problem—we’re creating a foundation for more intelligent, more coordinated healthcare delivery.
Patient Understanding and Access
Alarming reality: A disturbing statistic often gets overlooked in discussions about healthcare technology: 88% of discharge instructions are written at a level patients cannot understand.
This isn’t just an inconvenience—it’s a crisis. When patients leave the hospital confused about their care plan, medication schedule, or warning signs to watch for, the results are predictable and devastating: 30% return within 30 days, often with preventable complications.
This communication gap costs the healthcare system $52 billion annually in preventable readmissions. But the human cost is far greater. Imagine leaving the hospital after a serious illness, holding papers filled with medical jargon, trying to understand how to take care of yourself or a loved one. The anxiety and uncertainty patients face in these moments is a failure of our healthcare system—and one that technology, properly applied, could largely solve.
Complex challenge: The challenge goes far beyond just simplifying medical language.
Studies show that effective patient communication must be:
- Personalized to each patient’s health literacy level
- Culturally appropriate and contextually relevant
- Available in the patient’s preferred language
- Delivered at the right time and in the right format
- Consistently reinforced across the care journey
Currently, achieving even one of these goals is difficult. Achieving all of them simultaneously has been nearly impossible—until now.
Transforming Patient Communication
Vision timeline: Here’s how AI could transform patient communication within the next 3-5 years:
Imagine a patient named Maria being discharged after a complex heart procedure. As her doctor discusses discharge instructions, the AI system is not just documenting the clinical notes—it’s simultaneously creating a multi-level communication framework:
- For her cardiologist: a detailed clinical summary
- For Maria: clear, sixth-grade level explanations
- For her family: simple daily care instructions
- For her daily life: a medication schedule that accounts for her specific routine
The system’s cultural and linguistic adaptation goes far beyond simple translation. All materials are automatically generated in both English and Spanish, but more importantly, the instructions respect Maria’s cultural dietary preferences and reference support systems available in her community. The explanations use culturally relevant analogies and examples that resonate with her background and experience.
The follow-up system transforms from a static set of instructions to an interactive support network. Through a mobile app, Maria can:
- Ask questions about her recovery in plain language and receive clear, contextual answers
- Receive proactive reminders about medications and appointments
- Alert her care team if she reports concerning symptoms
- Maintain direct communication channels to her healthcare providers when needed
Technical Foundation and Real Challenges
Technical progress: The technology to enable this transformation is emerging rapidly.
Key capabilities include:
- Large language models can now reliably “translate” between medical and plain language
- Mobile devices can deliver personalized health information securely
- Natural language processing can understand and respond to patient questions with increasing sophistication
- AI can maintain context across entire episodes of care, ensuring continuity and coherence in communication
Healthcare Economics
The healthcare economy suffers from a peculiar inefficiency: we spend enormous resources documenting care, yet this documentation often fails to capture the true value and complexity of that care. This isn’t just an accounting problem—it’s a fundamental barrier to healthcare delivery and access.
Economic impact: Consider these stark realities:
- Hospitals lose approximately $52 billion annually to preventable readmissions
- Up to 40% of claims are initially denied, largely due to documentation issues
- The average hospital spends $8.6 million annually just on documentation-related activities
- Prior authorization delays cost hospitals about $40 million per year in delayed or lost revenue
Each statistic represents not just lost money, but lost opportunities to provide care.
What’s particularly striking is that this crisis is largely artificial. The value of healthcare isn’t actually declining—our ability to capture and communicate that value is simply failing to keep pace with its complexity. When a hospital loses money on a case, it’s frequently not because the care was inappropriate or inefficient, but because the documentation failed to properly reflect the care’s complexity and medical necessity.
Timeline perspective: I believe AI could fundamentally transform this aspect of healthcare within 5-7 years, but only if we understand what real transformation looks like.
It’s not just about better billing or faster claims processing. The goal isn’t to help hospitals make more money—it’s to ensure that resources flow efficiently to support optimal care delivery.
A Vision of Economic Transformation
Let me paint a picture of what this transformation might look like:
Imagine a hospital where economic decisions happen seamlessly alongside clinical ones, where documentation automatically captures not just what was done, but why it was necessary and valuable. As a doctor conducts rounds, the AI doesn’t just document clinical findings—it simultaneously:
- Recognizes complex medical conditions and their interactions
- Maps clinical scenarios to appropriate billing codes in real-time
- Identifies missing documentation needed for proper reimbursement
- Initiates prior authorization requests proactively
- Predicts and prevents likely claim denials
- Maintains continuous documentation of medical necessity
Key principle: Most importantly, this all happens without requiring clinicians to think about billing or coding.
The technology understands the economic implications of clinical decisions automatically, ensuring that legitimate care is properly valued and reimbursed.
Beyond Financial Efficiency
The impact extends far beyond just financial efficiency. Here’s what becomes possible:
Value-Based Care Implementation
When we can properly capture the full context and value of care, transitioning to value-based payment models becomes practical rather than aspirational. Quality metrics become natural byproducts of care documentation rather than separate reporting burdens.
Resource Optimization
Hospitals can move from reactive to proactive resource management, predicting needs based on population health trends and ensuring resources are available where they’re needed most.
True Price Transparency
Instead of generic price estimates, we can provide patients with personalized cost predictions that account for their specific clinical situation, insurance coverage, and potential complications.
Care Access Expansion
When hospitals can operate more efficiently, they can extend care to previously underserved populations and maintain services in communities that currently lack access.
Technical Capabilities
Current progress: The technical requirements for this vision are significant but achievable. We’re already seeing early versions of these capabilities:
- Natural language processing can understand complex clinical context
- Machine learning can predict claim denials with high accuracy
- AI can map clinical narratives to billing codes reliably
- Real-time integration with insurance systems is becoming possible
Healthcare Equity and Access
The innovations discussed so far—in documentation, patient communication, economics, and clinical decision support—could dramatically improve healthcare delivery. But a crucial question remains: will these improvements reach everyone? Healthcare disparities are stark and persistent. Rural Americans live an average of 10.5 miles from the nearest hospital, compared to 5.6 miles for urban residents. Nearly 25% of rural hospitals are at risk of closure. Language barriers affect care quality for over 20 million Americans with limited English proficiency. These aren’t just statistics—they represent millions of people receiving suboptimal care or no care at all.
But what’s particularly striking about healthcare disparities is that many are fundamentally issues of resource distribution and access, precisely the kind of challenges that AI could help address. While technology alone can’t solve structural inequality, it can help extend the reach and impact of existing healthcare resources.
Let me paint a picture of how AI could transform healthcare equity within 5-7 years:
The Reality of Healthcare Disparities
Critical context: The healthcare gap between urban and rural America isn’t just a statistic—it’s a daily reality for millions.
Rural Americans live an average of 10.5 miles from the nearest hospital, nearly twice the distance of their urban counterparts. Nearly 25% of rural hospitals are at risk of closure. Over 20 million Americans face care barriers due to limited English proficiency. These aren’t just numbers—they represent millions receiving suboptimal care or no care at all.
But what’s particularly striking about healthcare disparities is that many are fundamentally issues of resource distribution and access—precisely the kind of challenges that AI could help address. While technology alone can’t solve structural inequality, it can help extend the reach and impact of existing healthcare resources in ways that were previously impossible.
Transforming Access Through Technology
Vision example: Imagine a rural hospital in Montana.
Today, it struggles to maintain basic services. But with advanced AI systems, it becomes a hub of sophisticated care delivery. When a patient arrives with a complex condition, the AI doesn’t just transcribe the local doctor’s notes—it simultaneously coordinates with specialists across the country, providing real-time translation if needed, and synthesizing recommendations that account for both leading-edge medical knowledge and local resource constraints.
The system doesn’t just react to patients who make it to the hospital—it proactively identifies community health needs through analysis of population data, environmental factors, and social determinants of health. Mobile health units, guided by predictive analytics, bring care to those who need it most. The AI doesn’t just speak multiple languages—it understands cultural contexts, adapting health education and recommendations to align with local beliefs and practices.
Real-World Impact
But the impact goes far beyond individual hospitals. Here’s what becomes possible:
Cross-Cultural Care
When a Spanish-speaking grandmother in rural Texas needs specialized cardiac care, she no longer faces a choice between traveling hundreds of miles or settling for limited local options. The AI enables her local provider to deliver specialist-guided care in her native language, with cultural nuances preserved and respected.
Community-Specific Solutions
When a Native American community faces a diabetes crisis, the system doesn’t just flag the problem—it helps design interventions that respect traditional practices while incorporating modern medical knowledge. The AI learns from success stories in similar communities, adapting strategies that have worked elsewhere while maintaining sensitivity to local contexts.
Technical Foundation
Current capabilities: The technical requirements for this vision are significant but achievable. We’re already seeing early versions of these capabilities:
- Neural translation models achieving near-human accuracy in medical contexts
- Population health models predicting community needs with increasing precision
- Mobile health platforms delivering sophisticated care in resource-limited settings
But the real challenges aren’t primarily technical. They involve:
- Building sustainable infrastructure in remote areas
- Earning trust in communities with historical reasons for skepticism
- Navigating complex regulatory requirements
- Securing long-term funding commitments
Implementation timeline: This is why I believe a 7-10 year timeline is realistic but not conservative.
The core technology might be ready sooner, but full implementation requires careful attention to these human and systemic factors. The path forward isn’t simple, but the stakes couldn’t be higher. When we talk about healthcare equity, we’re not just discussing abstract principles—we’re talking about fundamental human dignity and the right to health.
Measuring Success
Success in healthcare equity requires clear metrics:
- Reduction in care disparities between populations
- Improved health outcomes in underserved areas
- Increased access to preventive services
- Better management of chronic conditions
- Higher patient satisfaction across all communities
Yet the real measure of success goes beyond statistics. True healthcare equity means that every person, regardless of location, language, or socioeconomic status, has access to high-quality, culturally appropriate care. AI can help make this vision achievable by extending the reach of healthcare resources and ensuring consistent quality across all settings.
Core mission: This isn’t just about making healthcare more efficient—it’s about fulfilling healthcare’s fundamental promise of caring for all people.
When we can truly deliver quality care everywhere, we don’t just improve health outcomes—we strengthen the social fabric of our communities and uphold the basic dignity of every person.
Addressing Common Concerns
Throughout discussions of healthcare AI, I often encounter a predictable set of concerns. What’s striking isn’t just how consistent these concerns are, but how deeply they reflect healthcare professionals’ hard-won wisdom about technology. Each objection carries the weight of past disappointments—from EHR implementations that increased workload rather than reducing it, to clinical decision support systems that generated more noise than signal.
Key Concerns and Responses
Foundational concern: The most frequent objection I hear is that AI will replace clinical judgment.
This reveals a profound misunderstanding of AI’s role in healthcare. Consider how physicians already use technology: A stethoscope doesn’t replace a doctor’s ability to diagnose—it amplifies it. A radiologist’s report doesn’t replace clinical reasoning—it informs it. AI should function similarly, amplifying rather than replacing human expertise.
The data supports this complementary approach. A 2023 study particularly caught my attention: When AI and physicians worked together, diagnostic accuracy reached 98%, significantly higher than either physicians (92%) or AI (89%) alone. What’s especially striking is that patient satisfaction actually increased when AI supported rather than replaced human interaction.
Understanding Medical Context
Technical reality: Concerns about voice AI’s ability to understand medical context stem from painful experience with earlier systems.
Many clinicians remember struggling with voice recognition that couldn’t handle basic medical terminology, let alone complex clinical context. But recent advances have fundamentally changed what’s possible. Modern systems achieve 99% accuracy in medical terminology and can maintain context across entire patient encounters. More importantly, they’re designed to fail safely—explicitly flagging uncertainty rather than making dangerous assumptions.
Privacy and Security
Privacy concerns reflect healthcare’s fundamental ethical obligations. Healthcare privacy isn’t a nice-to-have feature—it’s a core requirement built into the profession’s DNA. Any voice AI system must meet extraordinary security standards, from end-to-end encryption to HIPAA compliance. But security goes beyond technical specifications. It requires building systems that earn trust through transparent operation and clear accountability.
Healthcare Disparities
Critical consideration: The worry that AI might increase healthcare disparities deserves particular attention.
Technology often does benefit privileged populations first. But I believe AI presents a unique opportunity to reverse this pattern. By reducing documentation costs and improving efficiency, we can extend care to underserved areas. By supporting multiple languages and cultures, we can break down barriers that have long limited access to quality care.
Technology Dependence
The specter of technology dependence looms large, especially given healthcare’s experience with EHR outages. Any new system must include:
- Robust fallbacks
- Graceful degradation capabilities
- Enhancement rather than replacement of core clinical competencies
Medical Education Impact
Some worry about AI’s impact on medical education. Won’t residents become dependent on AI assistance? But this concern misunderstands how learning happens in medicine. Just as the calculator didn’t prevent students from understanding mathematics, AI won’t prevent residents from developing clinical judgment. In fact, by reducing administrative burden, AI could allow more time for direct patient interaction and clinical learning.
Key insight: These concerns aren’t just obstacles to overcome—they’re guideposts for responsible implementation.
From Vision to Reality: The Technical Foundations
While much of our discussion has focused on the transformative potential of AI in healthcare, it’s crucial to understand the technical foundations that make this transformation possible. The gap between inspiring visions and practical implementation often comes down to solving specific technical challenges. Let me outline how we’re bridging this gap through OrbNet’s development.
The Technical Architecture of Change
Core challenge: The fundamental challenge in healthcare transformation isn’t just developing new technologies—it’s developing technologies that work within healthcare’s unique constraints.
Our technical approach centers on three core innovations that directly address these constraints:
1. Edge-Optimized Intelligence
Traditional approaches to healthcare AI have relied on centralized processing, creating latency that makes real-time clinical support impossible. Through specialized compression techniques and depthwise convolutional networks, we’ve achieved sub-500 millisecond response times on edge devices. This isn’t just a technical metric—it’s the difference between reactive and proactive care.
Real-world impact: Consider what this means in practice: When a clinician is assessing a patient, they don’t need to wait for server responses or deal with connectivity issues.
The intelligence is right there, processing in real-time, adapting to the specific clinical context. This transforms the nature of clinical decision support from a reference tool to an active partner in care delivery.
2. Multimodal Integration
Healthcare data doesn’t exist in neat, standardized packages. It comes from multiple sources, in various formats, often with critical gaps. Our FHIR-based integration pipeline doesn’t just collect this data—it makes sense of it. Through advanced sensor fusion techniques and graph neural networks, we can identify subtle patterns that would be impossible to spot in fragmented data streams.
This technical capability enables a fundamental shift in care coordination. Instead of piecing together information from multiple systems, clinicians can see a complete, contextual picture of patient status. The technology fades into the background, letting healthcare providers focus on what matters: patient care.
3. Protected Innovation
Perhaps most crucially, we’ve developed these capabilities while maintaining strict security and privacy controls. Our patent-pending techniques for on-device learning and distributed analytics ensure that innovation never comes at the cost of patient privacy. This isn’t just about compliance—it’s about building technology that upholds healthcare’s fundamental values.
From Technical Capability to Healthcare Transformation
These technical innovations enable the broader transformation we’ve discussed throughout this article. By solving specific technical challenges around edge computing, data integration, and privacy protection, we create the foundation for reimagining healthcare delivery.
Consider how these capabilities support the key transformations we’ve outlined:
Clinical Communication and Documentation
- Real-time processing enables truly ambient clinical documentation
- Multimodal integration ensures complete capture of clinical context
- Edge optimization allows seamless mobile use
Patient Understanding and Access
- Protected innovation enables secure sharing of personalized insights
- FHIR standardization supports interoperability across care settings
- Edge processing enables responsive patient interfaces
Healthcare Economics
- Comprehensive data capture supports accurate billing and coding
- Real-time processing enables proactive resource management
- Protected innovation ensures compliance with regulatory requirements
Technical Evolution and Future Development
Development status: The technical readiness level we’ve achieved (TRL 3) indicates that these aren’t just theoretical possibilities—they’re practical innovations on the path to deployment.
While challenges remain, particularly around regulatory clearance and scaling, the core technical foundations are solid.
Looking Ahead: Technical Evolution and Healthcare Transformation
As we continue developing these technologies, we’re focused on several key areas:
Enhanced Clinical Intelligence
- Further optimization of edge-based neural networks
- Expanded pattern recognition capabilities
- Improved real-time decision support
Seamless Integration
- Broader FHIR-based interoperability
- Enhanced multimodal data fusion
- Streamlined workflow integration
Advanced Protection
- Expanded privacy-preserving techniques
- Enhanced security controls
- Improved consent management
These technical advances will enable the next wave of healthcare transformation, supporting the vision we’ve outlined while ensuring practical implementation in real clinical settings.
Path forward: The path from technical innovation to healthcare transformation isn’t straightforward, but it’s one we must navigate thoughtfully.
By grounding our transformative vision in solid technical foundations, we can turn the promise of AI-enabled healthcare into reality—not just as a technical achievement, but as a fundamental improvement in how we deliver care.
Conclusion: The Moral Imperative
Throughout this essay, I’ve outlined a vision of transformed healthcare that might seem ambitious or even unrealistic to some. Yet I believe this transformation isn’t just possible—it’s morally necessary. Every day we delay implementing these capabilities means:
- More preventable medical errors harming patients
- More provider time lost to administrative burden
- Wider disparities in care affecting vulnerable populations
- Persistent knowledge gaps compromising treatment decisions
Core mission: Healthcare’s fundamental promise is to heal, to comfort, and to care for all who need help.
Today, despite the dedication of healthcare professionals, our system often fails to fulfill this promise. Not because we lack the knowledge or the will to help, but because our tools and systems haven’t kept pace with healthcare’s complexity.
The Opportunity Before Us
We have a unique opportunity—and therefore a moral obligation—to change this. The technologies we’re developing could help fulfill healthcare’s core mission: to provide the best possible care to every patient, regardless of their circumstances. This transformation won’t happen overnight, nor should it. True change in healthcare requires:
- Careful staging
- Clinical leadership
- Unwavering focus on patient outcomes
But with the right approach, we can begin making meaningful progress today while building toward our larger vision.
The Path Forward
The path forward isn’t simple. It requires:
- Careful attention to safety and privacy
- Thoughtful integration with existing systems
- Deep respect for healthcare’s human elements
- Unwavering commitment to equity and access
Success demands that we move thoughtfully but purposefully, building trust through demonstrated results while maintaining the highest standards of patient safety. But the difficulty of the journey doesn’t diminish its importance. In fact, the very challenges we face in implementing these changes remind us of their value.
Critical choice: The choice before us isn’t whether to embrace this transformation—it’s whether to shape it thoughtfully or let it happen haphazardly.
The technology will advance regardless of our choices. Our responsibility is to ensure it advances in ways that serve healthcare’s fundamental mission and values, guided by clinical wisdom and grounded in real-world practice.
This is why we must:
- Maintain an intense focus on safety and validation
- Work in close partnership with healthcare professionals
- Listen carefully to patient needs and concerns
- Never waver in our commitment to equity
By embracing a measured, clinically-led approach to transformation, we can ensure that each step forward strengthens rather than disrupts the practice of medicine.
Final Thoughts
Vision for the future: The future of healthcare isn’t just about technology—it’s about people.
It’s about:
- Giving providers the tools they need to practice medicine at its highest level
- Ensuring every patient receives the care they deserve
- Fulfilling the ancient promise of medicine: to heal, to help, and to offer hope
This transformation is our generation’s opportunity—and responsibility—to advance the timeless mission of healthcare. Let’s embrace it with the wisdom, care, and determination it deserves.