The Next Evolution of Healthcare AI: Why 2025 Marks the Shift from Tools to Teammates
February 12, 2025 | Abdus-Salaam Muwwakkil - Chief Executive Officer
Table of Contents
- 1. The Surprising Limits of Human-AI Collaboration
- 2. The Rise of AI Agents: A New Kind of Healthcare Partner
- 3. The Teammate Model: Rethinking Human-AI Relationships
- 4. Implementing the Future: Challenges and Solutions
- 5. The Path Forward: 2025 and Beyond
- Conclusion
- Key Takeaways (SEO-Friendly Highlights)
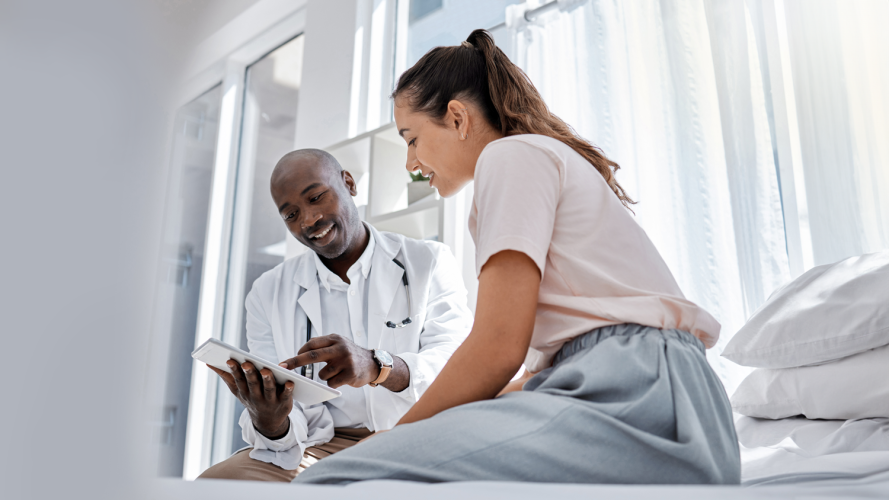
The Next Evolution of Healthcare AI: Why 2025 Marks the Shift from Tools to Teammates
In a quiet research lab at MIT, scientists recently made an unexpected discovery about healthcare artificial intelligence—one that is shaking our assumptions about how best to integrate AI with clinical practice. When doctors worked alongside a high-performing AI system to detect abnormalities in chest X-rays, the combined accuracy turned out to be lower than that of the AI working alone. The implications are hard to ignore: the conventional wisdom that “human plus machine” naturally trumps either working solo does not always hold true.
Far from being a one-off anomaly, this surprising result appears across multiple studies, from Harvard to large-scale European trials. Yet these findings do not suggest we abandon AI altogether—on the contrary, they point to an evolving relationship between clinicians and AI that is poised to transform healthcare over the next few years. As we head into 2025, a pivotal shift is underway: AI is no longer just a passive tool for automated tasks but is emerging as a proactive teammate in patient care.
Below, we unpack why some AI systems work better independently, how the next generation of “AI agents” will augment healthcare, and what it takes for humans and machines to genuinely collaborate as teammates. We’ll explore three critical collaboration models, the infrastructure and governance challenges ahead, and how an effective “teammate model” can re-humanize clinical practice—restoring time and empathy to overburdened healthcare professionals.
1. The Surprising Limits of Human-AI Collaboration
A Radiology Riddle
Imagine a radiologist examining chest X-rays with assistance from a state-of-the-art AI algorithm. Common sense suggests their partnership should be unbeatable: the physician contributes years of experience and nuanced clinical judgment, while the algorithm offers lightning-fast image processing and pattern recognition.
But in trials at MIT and Harvard-affiliated hospitals, doctors who saw AI predictions performed worse than the AI operating on its own. Accuracy rates were telling:
- AI alone: 92% accuracy
- Doctors alone: ~74% accuracy
- Doctors + AI together: Only 76% accuracy
Why does this happen? Researchers point to “automation neglect,” where humans undervalue or dismiss AI input, especially if it conflicts with their initial impression. Another factor is cognitive disruption: clinicians are accustomed to certain diagnostic workflows, and AI-generated suggestions can create extra steps or “analysis paralysis,” ultimately degrading performance.
The Swedish Mammogram Revelation
A landmark Swedish study of more than 80,000 mammograms split participants into two groups:
- Traditional Protocol: Each scan assessed by two radiologists.
- AI-First Approach: AI performed the initial screening, flagging suspicious cases for targeted human review only when necessary.
The results were striking:
- The AI-first method identified 20% more breast cancers.
- Radiologist workload dropped by nearly 50%.
This outcome underscores a vital lesson: sometimes separating tasks produces better results than forced collaboration. Letting AI carry out the preliminary screening—rather than having humans constantly second-guess AI outputs—improved both accuracy and efficiency.
2. The Rise of AI Agents: A New Kind of Healthcare Partner
From Reactive Tools to Proactive Agents
For the past decade, AI in healthcare has been primarily reactive. Algorithms functioned like advanced calculators: data in, output out, then back to idle. A new generation of AI—often termed “AI agents”—is changing that dynamic entirely. These agents are:
- Continuously Aware: Monitoring patient data in real-time, from vitals to wearable trackers.
- Proactive: Issuing alerts or initiating next steps if they detect anomalies or concerning trends.
- Context-Sensitive: Synthesizing data from imaging, labs, and EHR notes to adapt recommendations over time.
- Autonomously Learning: Improving accuracy and workflows through repeated interactions with clinicians and patient outcomes.
According to Dennis Chornenky, chief AI adviser at UC Davis Health, these agents “don’t just respond to queries; they maintain ongoing awareness of patient care.” Picture an AI system that not only transcribes a clinic visit but:
- Flags medication contraindications or duplications.
- Suggests follow-up appointments based on patient history and clinical guidelines.
- Alerts specialists when their expertise might be needed.
- Checks whether a patient has picked up prescriptions or followed physical therapy routines.
Implications for Healthcare Delivery
These proactive capabilities can dramatically reduce administrative overhead—such as verifying orders or chasing down incomplete charts—and also fill care gaps by spotlighting urgent concerns in near-real time. However, this autonomy raises safety, governance, and liability questions. What if an AI agent orders an incorrect test? Who ensures it’s verified?
Leading medical centers are piloting AI agents for tasks like post-surgical recovery, where the agent tracks vitals, flags complications, and coordinates communication among care teams. Early evidence suggests that, when carefully overseen, AI agents can offer a powerful new paradigm for personalized, continuous care.
3. The Teammate Model: Rethinking Human-AI Relationships
Why “Teammate” Instead of “Tool” or “Replacement”?
The AI-in-healthcare debate often devolves into two extremes: AI as a tool to be controlled, or AI as a replacement for clinicians. In reality, neither approach realizes AI’s full potential. The best outcomes emerge when we treat AI as a teammate—an ongoing partnership where each entity does what it does best.
Humans shine at contextual reasoning, empathy, and creative problem-solving. AI excels at pattern recognition, continuous monitoring, and high-volume data handling. The challenge is deciding when and how to fuse these strengths without forcing awkward overlaps or ignoring synergy points.
Three Patterns of Effective Collaboration
-
Sequential Model
- Human first, AI second. Doctors excel at gathering patient information via interviews and physical exams. AI’s diagnostic accuracy can drop significantly—from 82% to 63%—if it tries to conduct interviews on its own. Once the human obtains the nuanced data, AI can analyze it for hidden patterns or risk scores, augmenting the doctor’s decision-making.
-
Collaborative Model
- AI first, then human refinement. Especially in imaging and large data sets, AI can rapidly triage findings and propose possible diagnoses. Physicians then apply clinical judgment, weighing comorbidities, patient preferences, and resource constraints to refine or override the AI suggestions.
-
Separation Model
- Independent task handling. As demonstrated in the Swedish mammogram study, AI can handle routine screenings, while human specialists step in only for flagged cases. Beyond imaging, this could apply to administrative tasks or prior authorizations—letting clinicians devote more time and mental energy to complex clinical scenarios that demand human empathy and advanced problem-solving.
4. Implementing the Future: Challenges and Solutions
Adopting AI “teammates” in healthcare isn’t about flipping a switch. Hospitals, clinics, and health systems must address infrastructure, safety governance, and workforce development so that AI genuinely enhances patient care.
4.1 Technical Infrastructure
Robust Data Integration
- AI agents depend on holistic, real-time data—requiring seamless integration between EHRs, lab systems, imaging platforms, pharmacies, and potentially patient wearables.
- Interoperability is key. Legacy or siloed systems often make data flow cumbersome.
Reliable Communication & Security
- AI teammates need user-friendly physician interfaces (dashboards, mobile apps) and encrypted channels to safeguard patient data.
- Cyberattacks can cripple AI systems if they access large troves of sensitive information. Vigilant security protocols are non-negotiable.
4.2 Safety Governance
Clear Protocols for AI Autonomy
- As AI takes more initiative, healthcare organizations must define which tasks require human sign-off.
- “AI-to-AI interactions” are on the horizon—one system may order confirmatory tests after another flags an abnormality. Institutions need guardrails akin to drug–drug interaction checks.
Performance Monitoring and Audits
- AI systems require continuous quality checks, akin to monitoring other high-stakes medical devices.
- Alert fatigue can be just as dangerous as missed alerts. Real-time analytics should track both AI’s standalone accuracy and the human-AI combined performance to ensure synergy, not interference.
4.3 Workforce Development
AI Literacy for Clinicians
- Physicians and staff need a baseline data science understanding: how AI learns, what biases can creep in, and when to suspect algorithmic error.
- Training should tackle myths (e.g., AI is always correct) and emphasize realistic capabilities vs. limitations.
Collaboration Skills & Workflows
- Mastering “communication” with a non-human teammate demands new clinical workflows—protocols for reconciling disagreements or escalating complex cases.
- Organizational culture should encourage clinicians to see AI as an ally rather than a threat.
Addressing Burnout and Cultural Resistance
- Properly designed AI can reduce administrative burdens, letting doctors spend more time on direct patient care.
- If marketed only as an efficiency tool, clinicians may resist. Leadership must highlight the human benefits—how AI frees up empathy, creativity, and deeper patient connections.
5. The Path Forward: 2025 and Beyond
A New Era of Healthcare Delivery
Over the next few years, as AI agents and refined collaboration models mature, 2025 could mark a tipping point. From imaging to triage to chronic disease management, AI’s role may become a new standard of care. Health organizations ready to adapt will deliver more precise, efficient, and patient-focused medicine, gaining a competitive advantage in an era demanding value-based results.
Re-Humanizing Medicine
Paradoxically, integrating AI as a teammate might re-humanize healthcare:
- Empathy and Connection: Offloading repetitive or routine tasks to AI frees clinicians to focus on deeper patient engagement and relationship-building.
- Creative Problem-Solving: With AI handling raw data crunching, humans can tackle ethical nuances, comorbidities, and social determinants of health that defy algorithmic shortcuts.
- Narrative and Storytelling: Clinicians can reclaim the art of medicine—listening to patient stories, applying empathy, and forming holistic care plans—rather than scrambling through EHR interfaces.
When executed thoughtfully, AI teammates can actually reduce burnout by eliminating the “data clerk” aspects of medicine. Clinicians practice at the top of their license, forging stronger patient connections.
How to Get There
-
Policy and Regulation
- Governments and medical boards must update certification and licensing frameworks to reflect AI’s evolving roles.
- Transparent policies around AI usage, data privacy, and accountability are crucial.
-
Medical Education Overhaul
- From medical school to residency, trainees should confront AI-driven case studies and learn to scrutinize algorithmic outputs.
- Curricula must incorporate AI literacy and best practices for “teammate” workflows.
-
Multidisciplinary Collaboration
- Data scientists, clinicians, ethicists, cybersecurity experts, and human-factors engineers should co-create AI solutions.
- Human-factors design helps ensure AI fits naturally into clinical workflows.
-
Cultural Change
- Hospital leaders should champion AI’s potential to restore humanity in healthcare rather than framing it as mere automation.
- Open communication about successes, failures, and improvements fosters trust and adoption.
Conclusion
The fact that AI sometimes outperforms combined human–machine teams does not mean humans should step aside. Rather, it illuminates how vital it is to structure these partnerships effectively. The future of medicine depends on harnessing each party’s strengths: AI’s limitless computational power and pattern recognition, alongside the empathy, creativity, and contextual reasoning of human clinicians.
By 2025, these technologies and collaborative frameworks may reach a tipping point, altering how we diagnose illness, triage patients, and orchestrate care. Healthcare organizations that prepare today—investing in technology, governance, and educational reforms—will be the ones delivering better clinical outcomes, alleviating provider burnout, and forging a healthcare landscape that feels more personal than ever.
In short, the next chapter of healthcare AI is about more than better algorithms; it’s about reframing AI as a trusted teammate—one that helps doctors and nurses focus on what truly matters: caring for patients as whole people, not just data points. Embracing this vision of collaborative intelligence opens the door to a future that is simultaneously more efficient, more accurate, and more profoundly human.
Key Takeaways (SEO-Friendly Highlights)
- AI Alone vs. AI + Clinicians: Studies reveal combined teams can underperform if not integrated properly. AI alone may achieve 92% accuracy, while “AI + human” sometimes slips to 76%.
- Swedish Mammogram Study: Over 80,000 mammograms showed that AI-led screenings detected 20% more cancers while halving radiologist workload.
- AI Agents: These systems are proactive, continuously aware, and autonomously learning, moving beyond the reactive “tool” stage.
- Collaboration Models: The sequential, collaborative, and separation approaches can optimize human–AI teamwork.
- Implementation Challenges: Robust data integration, safety governance, and clinician training are critical for successful AI adoption.
- 2025 Milestone: Expect widespread acceptance of AI teammates in imaging, triage, chronic disease management, and more.
- Re-Humanizing Healthcare: By offloading routine tasks, AI can free clinicians for empathy, creativity, and deeper patient relationships, helping reduce burnout and improve patient satisfaction.